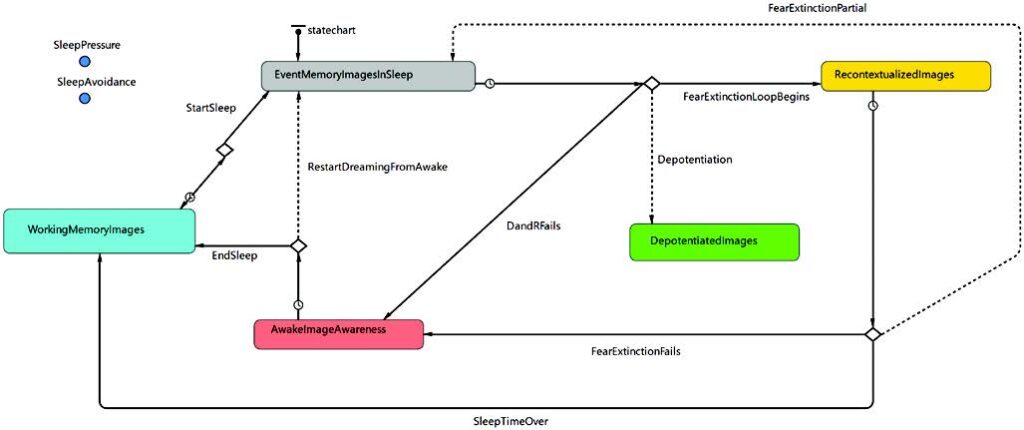
This is an article I published in 2021 (I’m a just a liiittle behind with updates on WW.com, but I’ll be making an effort to catch up in the coming weeks!) with neuroscientist Patrick McNamara and two colleagues from The Center for Mind and Culture, George Hodulik and David Rohr. The article presents a computational simulation of a prior conceptual model of disturbed dreaming published in 2007 by Levin and Nielsen. This publication builds on a prior pilot study using ReScript virtual reality technology to help people suffering from frequent nightmares to gain a sense of control over the frightening images that populate their nightmares. And it leads into the work we’re currently doing examining nightmare disorder among people who are 65 years and older. Eventually, we hope to use ReScript technology to help these elderly nightmare sufferers. Since older people often don’t have the visualization capacities needed to benefit from imagery rehearsal therapy – at present, the most effective non-pharmaceutical treatment for nightmare disorder – ReScript might prove an especially promising therapy for this underserved demographic.
Here’s a link to the full article; the abstract is included below. Check it out and leave a comment if you’re curious about the project!
Study Objectives: To test and extend Levin & Nielsen’s (2007) Affective Network Dysfunction (AND) model with nightmare disorder (ND) image characteristics, and then to implement the extension as a computational simulation, the Disturbed Dreaming Model (DDM).
Methods: We used AnyLogic V7.2 to computationally implement an extended AND model incorporating quantitative effects of image characteristics including valence, dominance, and arousal. We explored the DDM parameter space by varying parameters, running approximately one million runs, each for one month of model time, varying pathway bifurcation thresholds, image characteristics, and individual-difference variables to quantitively evaluate their combinatory effects on nightmare symptomology.
Results: The DDM shows that the AND model extended with pathway bifurcations and image properties is computationally coherent. Varying levels of image properties, we found that when nightmare images exhibit lower dominance and arousal levels, the ND agent will choose to sleep but then has a traumatic nightmare, whereas, when images exhibit greater than average dominance and arousal levels, the nightmares trigger sleep-avoidant behavior, but lower overall nightmare distress at the price of exacerbating nightmare effects during waking hours.
Conclusions: Computational simulation of nightmare symptomology within the AND framework suggests that nightmare image properties significantly influence nightmare symptomology. Computational models for sleep and dream studies are powerful tools for testing quantitative effects of variables affecting nightmare symptomology. The DDM confirms the value of extending the Levin & Nielsen AND model of disturbed dreaming/ND.